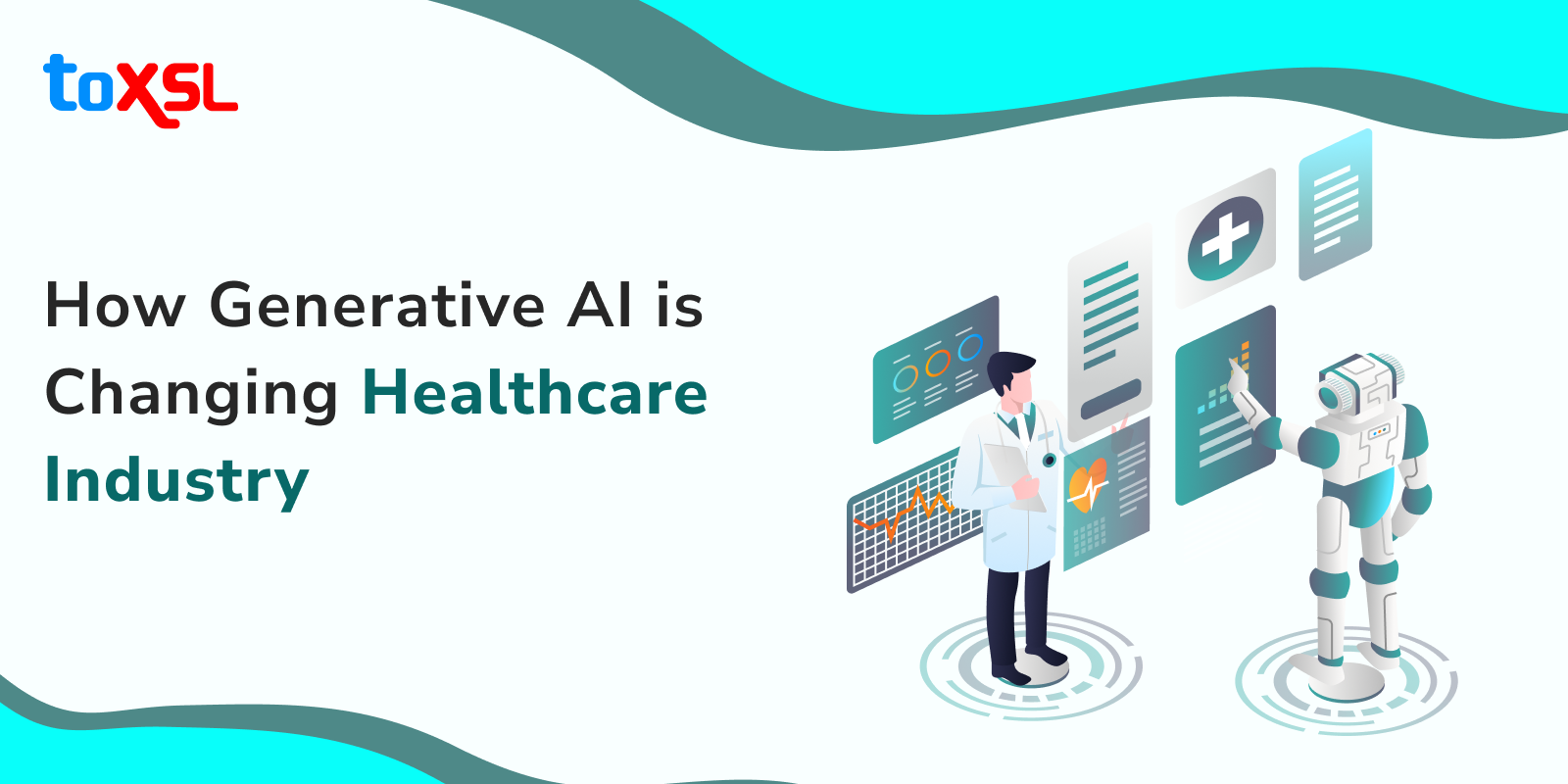
Generative AI, a subset of artificial intelligence, has been gaining significant attention in various industries, including healthcare. The potential of generative AI to revolutionize healthcare is vast and far-reaching. From improving diagnostic accuracy to enhancing patient engagement, the impact of generative AI on healthcare is likely to be profound. In this blog post, we will explore the current state of generative AI in healthcare, its statistics, potential applications, and challenges.
Statistics: Generative AI in Healthcare
Generative AI in healthcare has been gaining significant attention and investment in recent years. Here are some statistics on the current state of generative AI in the healthcare sector:
Market size: According to MarketsandMarkets, the global generative AI market in healthcare is projected to reach $3.4 billion by 2025, growing at a CAGR of 46.9% from 2020 to 2025.
Patient data analysis: Generative AI can analyze patient data such as medical images, clinical notes, and genomic data to identify patterns and make predictions. For instance, a study published in the journal Radiology used generative AI to analyze brain MRI scans and identified subtle changes in brain structure that could be indicative of neurodegenerative diseases.
Drug Discovery: Generative AI can create new drug candidates by modeling the chemical structures of known drugs and identifying potential leads. For example, a study published in the journal Nature used generative AI to design novel drugs that could potentially treat COVID-19.
Personalized Medicine: Generative AI can analyze genomic data and medical history to create personalized treatment plans for patients.
Medical Imaging Analysis: Generative AI can analyze medical images such as X-rays and MRIs to identify abnormalities and make diagnoses. For example, a study published in the journal Radiology used generative AI to analyze chest X-rays and identify potential lung cancer cases with high accuracy.
Remote Patient Monitoring: Generative AI can monitor patients remotely by analyzing data from wearable devices and other sources. For example, a study published in the journal JAMA used generative AI to monitor heart rate and blood pressure remotely in patients with hypertension.
Clinical Decision Support: Generative AI can provide clinical decision support by analyzing medical data and providing recommendations for diagnosis and treatment. For example, a study published in the journal Journal of Biomedical Informatics used generative AI to provide clinical decision support for diagnosing diabetes based on patient data.
Public Health Surveillance: Generative AI can monitor public health trends by analyzing data from various sources, such as electronic health records, social media, and news articles. For example, a study published in the journal PLOS Medicine used generative AI to monitor influenza outbreaks in real time.
Medical Writing: Generative AI can generate medical reports and summaries by analyzing data from electronic health records and other sources. For example, a study published in the journal Journal of the American Medical Association used generative AI to generate clinical notes based on patient data.
Ethical Considerations: Generative AI in healthcare raises ethical concerns such as data privacy, bias, and transparency. For example, a study published in the journal Nature Medicine discussed the ethical challenges of using generative AI in healthcare.
Impact of Generative AI in Healthcare: Applications
Let us now explore the impact of generative AI in healthcare and how it can improve patient outcomes, reduce costs, and enhance the overall quality of care.
1. Diagnosis and Treatment:
Generative AI can help doctors diagnose diseases more accurately and quickly than ever before. By analyzing medical images such as X-rays and MRIs, generative AI can identify tumors, lesions, and other abnormalities with a high degree of accuracy. This can lead to earlier detection and treatment, which can improve patient outcomes.
For example, researchers at the University of California, Los Angeles (UCLA) have developed an AI system that can analyze medical images to detect breast cancer with a high degree of accuracy. The system uses a deep learning algorithm to identify tumors and other abnormalities in mammography images, which can help doctors make more accurate diagnoses.
In addition to diagnosis, generative AI can also be used to develop personalized treatment plans for patients. By analyzing a patient's medical history, genetic data, and other factors, generative AI can suggest the most effective treatments and drugs for that particular patient. This can lead to more targeted and effective treatments, which can improve patient outcomes.
2. Drug Discovery:
Generative AI can also help researchers develop new drugs and therapies. By analyzing large amounts of data on the molecular structure of drugs, generative AI can suggest new drug candidates that could be effective in treating certain diseases. This can reduce the time and cost associated with traditional drug discovery methods, which often involve trial and error.
For example, researchers at the University of Toronto have developed an AI system that can design new drugs to treat diseases such as cancer and Alzheimer's. The system uses a generative model to predict the molecular structure of potential drug candidates, which can then be tested for effectiveness in clinical trials.
3. Patient Data Analysis:
Generative AI can also help analyze large amounts of patient data, such as electronic health records (EHRs) and medical imaging data. By identifying patterns and trends in this data, generative AI can help doctors make more accurate diagnoses and develop personalized treatment plans.
For example, researchers at the University of California, San Francisco (UCSF) have developed an AI system that can analyze EHRs to identify patients who are at high risk of developing certain diseases, such as diabetes or heart disease. The system uses a generative model to predict these risks based on patient demographics and medical history, which can help doctors intervene early and prevent the onset of these diseases.
4. Personalized Medicine:
Generative AI can also enable personalized medicine, which involves tailoring medical treatments to individual patients based on their unique genetic makeup and medical history. By analyzing large amounts of data on patient genetics and medical outcomes, generative AI can identify the most effective treatments for each patient.
For example, researchers at the University of California, Berkeley have developed an AI system that can analyze a patient's genetic data to predict their response to certain drugs. The system uses a generative model to identify the most effective drug combinations for each patient, which can lead to more targeted and effective treatments.
5. Medical Imaging Analysis:
Generative AI can also be used to analyze medical images such as CT scans and MRIs. By identifying patterns and abnormalities in these images, generative AI can help doctors diagnose diseases more accurately and quickly than ever before.
For example, researchers at the University of Utah have developed an AI system that can analyze medical images to detect signs of brain injury in patients. The system uses a generative model to identify abnormalities in MRI scans, which can help doctors diagnose and treat these injuries more effectively.
Generative AI: Challenges and Limitations
While generative AI has the potential to revolutionize healthcare, there are also challenges and limitations to consider. For example:
1. Data Quality and Availability: Generative AI models require high-quality and abundant data to learn and improve their performance. However, healthcare datasets are often incomplete, noisy, and inconsistent, which can negatively impact the accuracy of generative AI models. Moreover, data privacy and security concerns may limit the availability of sensitive medical data.
2. Explainability and Interpretability: Generative AI models are often opaque and difficult to interpret, making it challenging for clinicians to understand how the models arrive at their predictions or decisions. This lack of transparency can erode trust between clinicians and AI systems, hindering their adoption in healthcare.
3. Bias and Fairness: Generative AI models can perpetuate biases present in the training data, leading to unfair or discriminatory outcomes. For example, an AI system trained on a dataset with predominantly white patients may struggle to accurately diagnose or treat patients from diverse backgrounds. Ensuring fairness and equity in generative AI models is essential for their safe and effective deployment in healthcare.
4. Security and Privacy: Generative AI models can be vulnerable to cyberattacks, data breaches, and other security threats. Protecting patient data and maintaining confidentiality are critical concerns when implementing generative AI models in healthcare.
5. Human-AI Collaboration: While generative AI has the potential to augment clinical decision-making, it cannot replace human clinicians entirely. Building trust and facilitating effective collaboration between humans and AI systems is essential for optimizing healthcare outcomes.
6. Ethical Considerations: Generative AI models must be designed and deployed ethically, concerning patients' rights, autonomy, and dignity. Ensuring that AI systems prioritize patient well-being and avoid harmful actions or decisions is crucial in healthcare.
7. Clinical Validation: Generative AI models must be clinically validated to ensure their accuracy and effectiveness in healthcare settings. This requires rigorous testing and evaluation of the models against established clinical standards.
8. Integration with Existing Systems: Generative AI models must be integrated seamlessly into existing healthcare systems, including electronic health records (EHRs), laboratory information systems, and radiology systems.
9. Continuous Learning: Generative AI models require continuous learning and improvement to stay up-to-date with evolving medical knowledge and technologies. This necessitates ongoing investment in research and dartevelopment, as well as collaboration between academia, industry, and clinicians.
Conclusion
Generative AI has the potential to revolutionize healthcare by improving patient outcomes, reducing costs, and enhancing the overall quality of care. However, there are also challenges and limitations to consider, such as data quality and availability, regulatory and ethical issues, and integration with existing systems. As the use of generative AI in healthcare continues to grow, it is important to address these challenges head-on and ensure that this technology is used responsibly and effectively.
Frequently Asked Questions
1. What is Generative AI?
Ans: Generative AI refers to a subfield of artificial intelligence that focuses on creating or generating new content, such as images, videos, music, text, and even entire conversations. Generative AI models use machine learning algorithms to learn patterns in existing data and then generate new data that resembles the original but is not simply copied.
2. How does Generative AI work in healthcare?
Ans: Generative AI can be applied in various ways within the healthcare industry, such as image generation for medical imaging analysis, drug discovery and development, patient treatment plans, and even clinical trials. For instance, generative AI models could generate synthetic medical images that mimic real-world data but do not contain any personally identifiable information (PII), allowing for extensive training and testing without compromising patient privacy.
3. What are the benefits of Generative AI in healthcare?
Ans: Generative AI can improve healthcare efficiency, accuracy, and personalization. Here are some potential benefits of utilizing generative AI in healthcare:
1. Enhanced medical imaging analysis: Generative AI can create synthetic medical images that help train machine learning models for image recognition tasks, improving the accuracy of diagnosis and treatment.
2. Drug discovery and development: Generative AI can generate new molecular structures that could lead to novel drugs and therapies, streamlining the drug development process.
3. Personalized patient treatment plans: Generative AI models can create individualized treatment plans based on a patient's medical history, genetic markers, and lifestyle factors, resulting in more effective treatments with fewer side effects.
4. Improved clinical trials: Generative AI can simulate real-world scenarios to evaluate the effectiveness of new treatments or drugs without involving actual patients, reducing the need for expensive and time-consuming clinical trials.
5. Streamlined administrative tasks: Generative AI can automate routine administrative tasks within healthcare facilities, freeing up staff time for more critical tasks.
4. What are the challenges and limitations of Generative AI in healthcare?
Ans: Several challenges and limitations exist when implementing generative AI in healthcare, including:
1. Data quality and availability: Generative AI models require high-quality, diverse data to learn and generate accurate results. However, collecting and curating healthcare data can be complex due to privacy regulations and data fragmentation.
2. Explainability and interpretability: It can be challenging to understand the reasoning behind generative AI models' outputs, particularly when dealing with sensitive medical information. Developers must prioritize explainability and interpretability to ensure trustworthy results.
3. Regulatory compliance: Healthcare organizations must comply with strict regulations when implementing generative AI solutions. Ensuring that these solutions adhere to privacy and security standards is crucial.
4. Ethical considerations: Generative AI models can generate biased or offensive outputs if not properly trained on diverse data or monitored for ethical compliance. Developers must address these ethical concerns to avoid harming patients or perpetuating inequities.
5. How can we address these challenges?
Ans: Addressing the challenges and limitations of generative AI in healthcare requires a multifaceted approach that includes:
Collaboration between data scientists, clinicians, ethicists, and policymakers to ensure that generative AI solutions are not only technically proficient but also ethical and regulatory compliant.
Investing in research and development of generative AI models tailored to the healthcare industry's unique data environments and regulatory requirements.
Building a diverse and representative dataset that addresses privacy concerns while still providing adequate training data for generative AI models.
Developing techniques for explaining and interpreting the outputs of generative AI models, enabling clinicians to understand their decision-making processes and trust their conclusions.
Implementing robust quality control mechanisms to detect potential biases or inaccuracies in generative AI output, ensuring the safety and efficacy of healthcare services.
6. What is the future of Generative AI in healthcare?
Ans: The future of Generative AI in healthcare looks promising, with potential applications across various industries, including:
1. Personalized medicine: Generative AI models could help create individualized treatment plans based on a patient's genetic markers and medical history, leading to more effective treatments and better patient outcomes.
2. Drug discovery and development: Generative AI could accelerate drug discovery by generating new molecular structures with potential therapeutic properties, reducing the time and cost associated with traditional drug development methods.
3. Medical imaging analysis: Advanced generative AI models will improve image recognition tasks, enabling more accurate diagnoses and treatments for patients.
4. Public health surveillance: Generative AI could simulate real-world scenarios to evaluate the effectiveness of interventions or track disease transmission patterns, helping public health officials make informed decisions and improve outbreak response times.
5. Healthcare administration: Automating routine administrative tasks within healthcare facilities using generative AI could streamline operations and reduce staff workload.