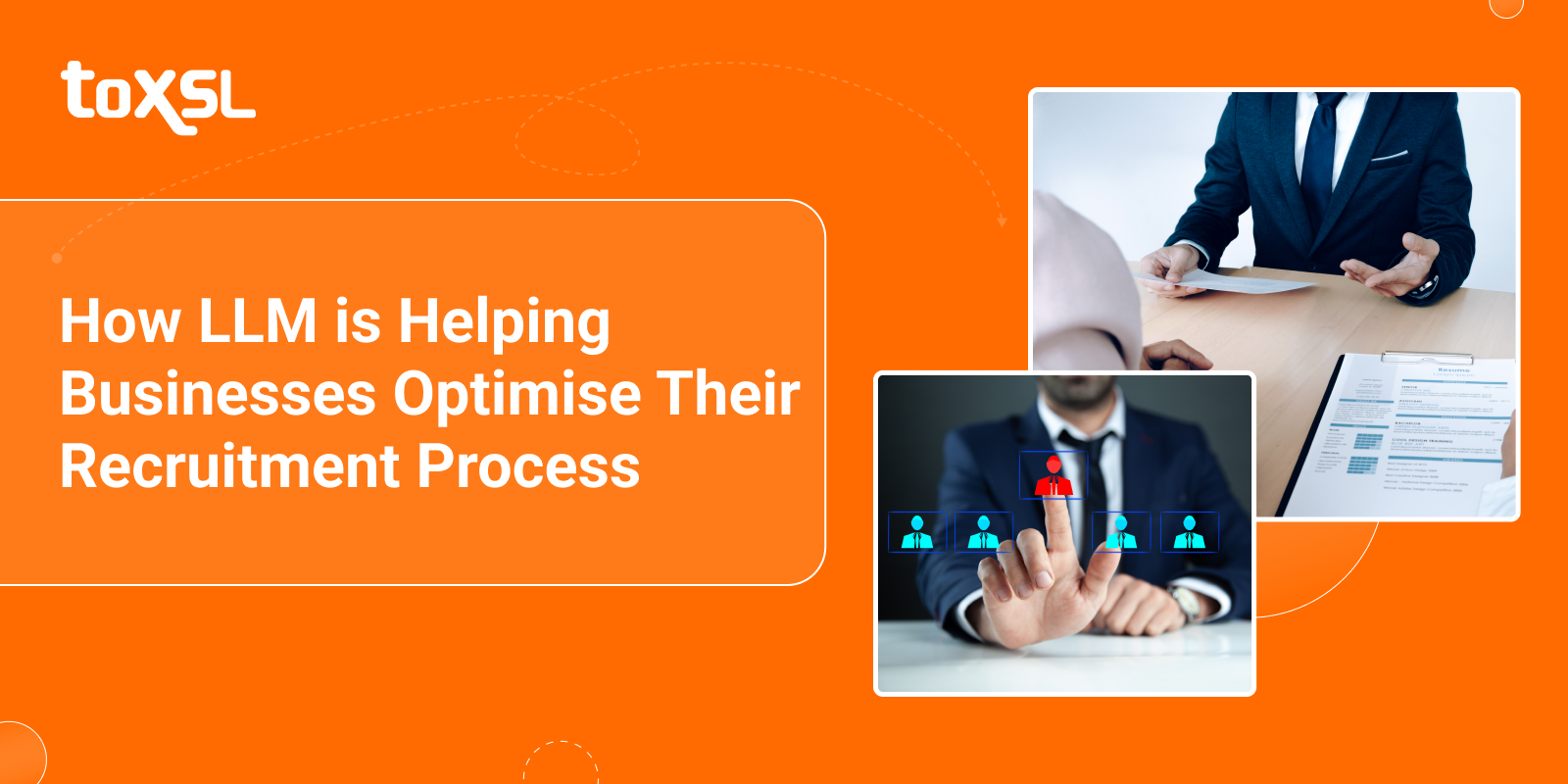
The world of hiring is rapidly changing, and technology is playing an increasingly important role in the process. One of the most exciting developments in this area is the rise of Large Learning Models (LLMs). These advanced AI-powered tools have the potential to revolutionize the way businesses hire by providing more accurate and efficient candidate selection processes.
Large Learning Models are machine learning models that use a large amount of data to train their algorithms. They are capable of handling complex tasks such as text classification, image recognition, and natural language processing. In the context of hiring, LLMs can analyze vast amounts of data to identify the most qualified candidates for a particular role. In this blog, let us explore how LLM can help you in hiring and the benefits it offers over traditional methods.
Benefits of Using Large Learning Models (LLMs) in Hiring:
Large Learning Models (LLMs) have gained significant attention in recent years due to their ability to process and analyze vast amounts of data, leading to improved decision-making across various industries. In the context of hiring, LLMs offer several benefits that can revolutionize the recruitment process and enhance the overall quality of talent acquisition.
This article explores the advantages of using LLMs in hiring and how they can positively impact organizations.
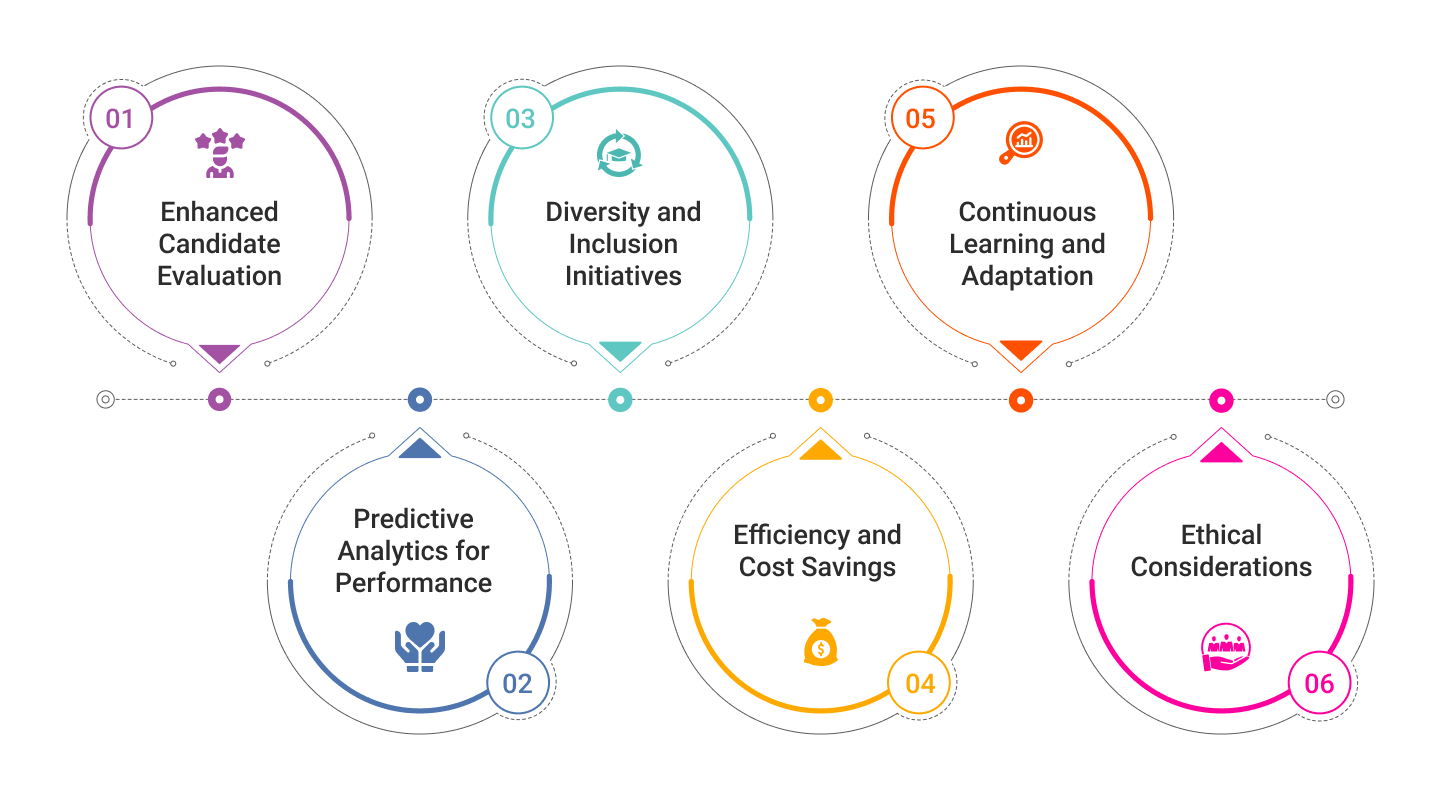
Enhanced Candidate Evaluation
One of the primary benefits of utilizing LLMs in hiring is the enhanced candidate evaluation process. Traditional hiring methods often rely on manual resume screening and interviews, which can be time-consuming and prone to biases. LLMs, powered by advanced algorithms and machine learning capabilities, can efficiently analyze a wide range of candidate data, including resumes, cover letters, social media profiles, and assessment results. By leveraging natural language processing and sentiment analysis, LLMs can extract valuable insights from unstructured data, enabling recruiters to gain a more comprehensive understanding of each candidate’s qualifications, skills, and cultural fit.
Predictive Analytics for Performance
Another compelling advantage of incorporating LLMs into the hiring process is the ability to leverage predictive analytics for assessing candidate performance. These models can analyze historical hiring data, employee performance metrics, and other relevant factors to identify patterns and correlations that contribute to successful job performance within specific roles or organizational contexts. By identifying these predictive indicators, organizations can make more informed decisions when selecting candidates who are likely to excel in their roles, ultimately reducing turnover rates and enhancing overall productivity.
Diversity and Inclusion Initiatives
LLMs have the potential to support diversity and inclusion initiatives within organizations by mitigating unconscious biases in the hiring process. Traditional methods of candidate evaluation may inadvertently perpetuate biases related to gender, ethnicity, or socioeconomic background. In contrast, LLMs can be trained to recognize and minimize such biases by focusing solely on relevant qualifications and competencies. Additionally, these models can help identify diverse talent pools that may have been overlooked through traditional recruiting methods, thereby promoting a more inclusive workforce.
Efficiency and Cost Savings
The use of LLMs in hiring can significantly improve efficiency and reduce costs associated with the recruitment process. Automated candidate screening and ranking capabilities offered by these models enable recruiters to handle large volumes of applications with greater speed and accuracy. Moreover, by streamlining initial candidate assessments, LLMs allow recruiters to allocate more time to strategic activities such as engaging with top-tier candidates and fostering employer branding efforts. Ultimately, this increased efficiency translates into cost savings for organizations by minimizing the resources required for manual screening and shortlisting processes.
Continuous Learning and Adaptation
LLMs are designed to continuously learn from new data inputs and adapt their algorithms based on feedback loops. In the context of hiring, this capability enables these models to evolve alongside changing job requirements, industry trends, and organizational needs. As a result, organizations can maintain a competitive edge in talent acquisition by leveraging LLMs that are adept at identifying emerging skill sets, industry-specific competencies, and evolving market demands.
Ethical Considerations
While the benefits of using LLMs in hiring are substantial, organizations must address ethical considerations associated with these technologies. Ensuring transparency in algorithmic decision-making processes, monitoring for potential biases in model outputs, and upholding data privacy standards are essential aspects of responsible LLM deployment in hiring practices.
Additional benefits of Large Learning Models (LLMs)
Improved Accuracy: LLMs can analyze large amounts of data to identify patterns and connections that may not be apparent to human recruiters. This means they can make more accurate predictions about a candidate's suitability for a role, reducing the risk of making a bad hire.
Time-saving: The automated nature of LLMs means they can process vast amounts of data much faster than humans. This means you can quickly identify the most qualified candidates and move them through your hiring process more efficiently.
Objective Decision Making: Unlike human recruiters, LLMs do not have personal biases or prejudices. They make decisions based solely on the data they are given, which can help reduce unconscious bias in your hiring process.
Challenges of Using Large Learning Models (LLMs) in Hiring
Large Learning Models (LLMs) have revolutionized the way organizations approach hiring, automating various aspects of the recruitment process and promising to increase efficiency and accuracy. However, these models also come with a set of challenges that need to be addressed to ensure they are effectively integrated into hiring practices.
This article explores the challenges of using LLMs in hiring and the steps that can be taken to overcome them.
Bias and Discrimination: LLMs are only as unbiased as the data they are trained on. If the data contains biases or stereotypes, the model will likely reproduce them. This can lead to discriminatory hiring practices that favor certain demographics over others, perpetuating existing inequalities in the workplace. To address this issue, organizations should ensure that their training data is diverse and representative of the population they aim to serve.
Privacy and Ethical Concerns: The use of LLMs in hiring raises concerns about the privacy of applicants’ personal information. Some models may require access to sensitive data, such as criminal records, political affiliations, or even health information. Organizations must be transparent about the data they collect and ensure that they only use it in ways that respect the privacy and dignity of applicants.
Cost and Resource Allocation: Implementing LLMs in hiring can be expensive, both in terms of the cost of the technology itself and the resources required to maintain and update the models. Organizations must carefully consider the potential return on investment and weigh the costs against the benefits of using these models in their hiring processes.
Legal Compliance: As the use of AI in hiring becomes more widespread, organizations must ensure that they comply with relevant labor laws and regulations. This may include addressing issues such as the prohibition of discriminatory practices, ensuring data privacy, and ensuring that applicants have the right to challenge automated decisions.
Interpretability and Explainability: One of the major challenges in using LLMs is their lack of interpretability and explainability. It can be difficult for organizations to understand how the model arrived at its conclusions, making it challenging to trust the results and incorporate them into their decision-making processes. Developing methods to interpret and explain the inner workings of LLMs is essential for their successful implementation in hiring.
Adaptability and Continuous Learning: As the landscape of hiring evolves, organisations must ensure that their LLMs can adapt to new situations and continue to learn from their experiences. This may involve regularly updating the models with new data, refining their algorithms, and developing new methods to assess candidates more effectively.
Conclusion
Large Learning Models (LLMs) offer a range of benefits for businesses looking to improve their hiring processes. By analyzing vast amounts of data, LLMs can identify the most qualified candidates quickly and efficiently, reducing the risk of making bad hires. However, there are also challenges to consider when using LLMs in hiring, such as data quality and interpretability. Overall, LLMs have the potential to revolutionize the way we hire by providing more accurate and efficient candidate selection processes. If you are looking to hire a company that can offer you amazing solutions using the LLM model, feel free to contact us. We at ToXSL Technologies have an amazing team of developers who are well-versed in Artificial Intelligence and all the innovation that is happening under the umbrella. Partner with us and see how our developers can help your business grow.
FAQ's
Q1. What is an LLM and how is it used in recruitment?
LLM (Large Language Model) is an AI tool that understands and generates human-like text. In recruitment, it helps screen resumes, match job descriptions with candidates, and even conduct initial interviews. This makes hiring faster, more accurate, and less time-consuming for HR teams.
Q2. How does LLM save time in the hiring process?
LLMs quickly scan resumes, shortlist candidates, and automate communication like interview scheduling and follow-ups. This reduces the manual effort of recruiters and speeds up the entire hiring cycle. Businesses can fill positions faster and reduce time-to-hire with the help of AI-driven tools.
Q3. Can LLM help find better candidates?
Yes, LLMs can analyze large data sets to find the most suitable candidates based on skills, experience, and job fit. They reduce human bias and ensure fair selection by using data-driven insights, helping businesses find the best talent for each role.
Q4. Is LLM recruitment technology easy to implement for small businesses?
Absolutely. LLM-based recruitment tools are scalable and easy to use. With support from experts like ToXSL Technologies, even small businesses can integrate AI into their hiring process, making recruitment smarter, quicker, and more efficient without needing a large HR team.